Data Warehouses: The Undying Titans of Information Storage
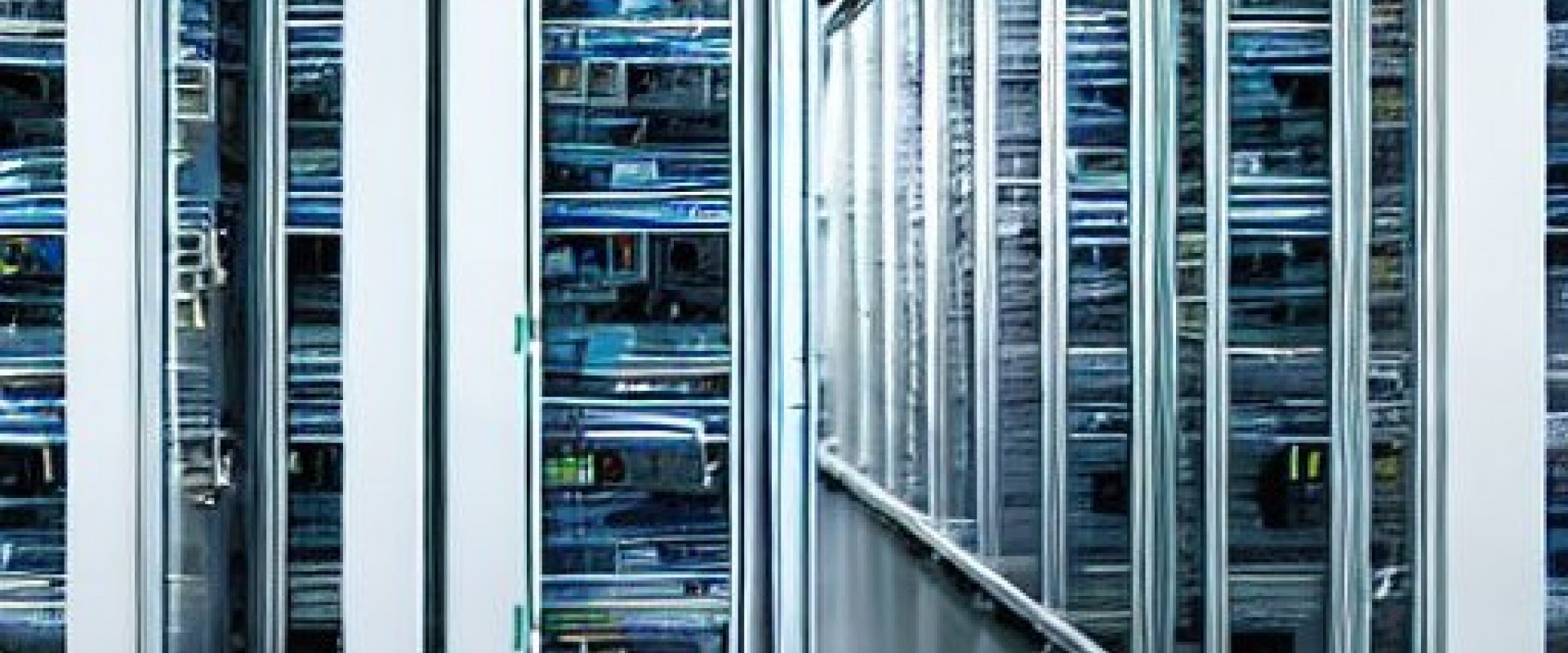
The data warehouses are now operating outside of the traditional IT infrastructure. The industry is constantly evolving, and there is no one-size-fits-all solution.
In the ever-evolving landscape of data management, the age-old rivalry between data warehouses and data lakes is finally being put to rest. It's no longer a matter of choosing one over the other; instead, it's about harnessing their combined power as a modern, integrated construct that benefits businesses and IT immensely. This blog post dives into data warehousing and sheds light on how it thrives as an undying titan of information storage.
First, we look at how data has become the driving force behind modern businesses. Understanding the significance and usage of the terms "data warehouse" and "data lake" forms the foundation of our exploration. By breaking down these concepts, we aim to bridge the gap between traditional and contemporary approaches, illustrating their symbiotic relationship in today's data-driven environment.
As we delve deeper, a simple yet powerful architectural model emerges, revealing how data warehouses and data lakes can coexist and complement each other. But the story doesn't end there; we also delve into three innovative architectural patterns, data fabric, data lakehouse, and data mesh, and examine their connection to this model. These emerging approaches open up exciting possibilities for collaborative data management, paving the way for more efficient and effective data operations.
Next, we shift our focus to ten specific areas where the strategic combination and placement of functionality and data across these two environments can optimize support for a multitude of business and technical needs. Organizations can unlock new opportunities for analysis, insights, and decision-making by finding the right balance between data warehouses and data lakes.
To bring our exploration to a satisfying conclusion, we shine a spotlight on the Cloudera Data Warehouse. This revolutionary solution encompasses the best of both worlds, seamlessly integrating traditional data warehousing and the flexibility of data lakes. Designed for modern digital business, this hybrid on-premises and multi-cloud platform offer a game-changing solution that empowers organizations to embrace the future while leveraging their existing data infrastructure.
Join me on this enlightening journey as we celebrate the endurance and adaptability of data warehouses in an era of ever-expanding information. Discover how these undying titans of information storage continue to shape the landscape of data management, providing organizations with the tools they need to thrive in a data-centric world.
From Data Marts To Data Lakes: The Evolution of Data Management
2010, a groundbreaking meme emerged, shaking up the data management world! introduced us to the data lake—a metaphorical reservoir of information in its natural, unprocessed state, contrasting the structured nature of data marts. The analogy struck a chord, resonating with its simplicity and memorability.
Enterprises at the forefront of innovation swiftly embraced the data lake, compelled by the technical imperative to leverage the vast influx of online big data streaming. The allure of cost savings through open-source software and commodity hardware further fueled their interest. In some cases, political motivations also played a role, as organizations sought to distance themselves from struggling data warehouse projects. Claims of replacing data warehouses with new data lakes proliferated throughout the decade, intensifying the debate between proponents of each approach.
As the cloud emerged as a dominant force in the market, architectural models and technology became erroneously entangled, leading to vague and often unstable implementations. Consequently, several data lakes became stagnant swamps, while others were eventually abandoned.
Amidst the ensuing confusion, a clarifying moment arrived in the form of a. It dispelled the notion of an either-or choice, emphasizing that data warehouses and data lakes are complementary concepts born from distinct business needs and technological possibilities. Data warehouses excel in providing accurate results for regulatory reporting and management decision-making, while data lakes enable exploration and innovation in fields like data science and machine learning.
Since then, a consensus has emerged, recognizing the inherent synergy between data lakes and data warehouses. Data and management processes should be shared between these entities, facilitated by advanced hybrid cloud technologies that now underpin most implementations. Consequently, the terminologies and meanings associated with lakes and warehouses have become intertwined. Analytical use cases traditionally delivered via data lakes now frequently leverage data warehouses.
However, ongoing challenges in implementation have given rise to three new architectural patterns: data fabric, data mesh, and data lakehouse. While proponents of each approach claim to offer the ultimate solution to data management issues, they possess distinct strengths and weaknesses. Furthermore, inconsistent terminology, varying definitions, and competing claims continue to sow confusion, further muddling basic data management concepts.
Thus, alongside questions about lakes and warehouses, additional queries arise. Is a fully decentralized approach to data management now imperative? Can artificial intelligence resolve long-standing metadata challenges? Is a unified technology base feasible or even desirable?
This blog ventures to provide answers to these complex questions, furnishing a solid foundation for contemplation. However, it recognizes multiple answers and options, contingent upon specific business needs and existing solutions, exist. Organizations can chart a course that aligns with their unique circumstances and aspirations by navigating the evolving data landscape.
The New Landscape of Data Warehouses: A New Breed of Beast
Data is no longer what it used to be. In the past, businesses could rely on a relatively small amount of structured data from their operational systems to make decisions. However, the rise of big data has changed all that. Today, businesses are generating more data than ever, coming from various sources, including social media, clickstreams, and the Internet of Things (IoT). This data is unstructured, and it is often in real-time. The data warehouses are now operating outside of the traditional IT infrastructure. The industry is constantly evolving, and there is no one-size-fits-all solution. This can make it difficult for businesses to choose the right data warehouse. They are often built on cloud-based platforms and use open-source software, which gives businesses more flexibility and control over their data. However, it also means data warehouses are more difficult to manage and secure.
The Challenges of Big Data
The challenges of big data are many. First, it isn't easy to manage. Traditional data warehouses were designed to store structured data, but big data is often unstructured. This makes it difficult to store, process, and analyze big data.
Second, big data is often in real-time. This means that businesses need to be able to analyze data as soon as it is generated. This can be a challenge for traditional data warehouses not designed for real-time analysis.
Third, big data is often used for predictive analytics. This means that businesses are using data to predict future behavior. This can be a powerful tool for businesses but also raises privacy concerns.
How Data Lakes Provide Modern State-of-the-art Storage Solutions
Data lakes are a new type of data storage solution designed to address big data challenges. Data lakes are designed to store all types of data, including structured, unstructured, and semi-structured data. This makes it easy to store and manage big data.
Data lakes are also designed for real-time analysis. This means that businesses can analyze data as soon as it is generated. This makes it possible for businesses to make faster, more informed decisions.
The Future of Data Warehousing
Data warehouses are not dead, but they are evolving. Traditional data warehouses are being replaced by hybrid data warehouses, which combine the benefits of data warehouses and data lakes. Hybrid data warehouses offer the best of both worlds. They can store all types of data, and they can be used for both historical analysis and real-time analysis.
Data is the new oil. In the digital age, data is a business's most valuable asset. Businesses that can collect, store, and analyze data will have a competitive advantage. Data lakes are a new type of data storage solution designed to address big data challenges. Data lakes are a key part of the future of data warehousing.
Combining the Competencies of a Data Warehouse and a Data Lake on an Enchanted Island
Combining a data warehouse and a data lake may appear straightforward, but they are distinct concepts. An analogy of a warehouse on an island in a lake can help illustrate how they complement each other and work together seamlessly in managing and utilizing data in a digital business.
While the conceptual definition of a data warehouse has remained largely stable over the past three decades, functional differences in design, such as Kimball's dimensional/star schema data model, still exist. The evolution of the concept has resulted in optimized components for specific purposes, driven by the changing characteristics of relational databases. The Enterprise Data Warehouse (EDW) plays a central role in differentiating between a data warehouse and a data lake, as it is responsible for cleansing and reconciling data from various operational sources.
The primary objective of a data warehouse is to provide reliable and consistent information to support decision-making, especially for legally relevant actions, performance tracking, and problem determination. It is important to note that a data warehouse contains more than raw data; it includes contextualized and cleansed information that has been prepared for valid and correct use. This detailed information can be further subdivided and summarized into appropriately structured data marts to enhance performance, ease of use, or security for business users.
Data in a warehouse or data marts primarily originates from operational systems, both traditional on-premises and modern web-based varieties. Other sources may also be included as long as the data meets quality standards and can be contextualized into useful and usable information. For instance, data in a data lake can be ingested into a warehouse through a cleansing and reconciliation process based on agreed data governance rules.
In contrast, a data lake is characterized by its ability to collect a wide range of data items without prior structuring into a preferred model. It is a multi-structured, often distributed data store that enables ingestion, processing, formatting, and management of high-volume raw data from multiple external sources. The data lake can fulfill diverse business and technical needs, including those covered by existing systems. However, it is important to note that the scope of a data lake should be realistic and practical rather than overly utopian.
The distinct characteristics and uses of data warehouses and data lakes have traditionally led to creating of separate technology implementations and silos of disconnected data. However, understanding their differences allows for creating an integrated architectural pattern that eliminates silos. Figure 1 illustrates this pattern, positioning the data warehouse and the data lake concerning each other and operational systems, facilitating comprehension by both business and IT stakeholders.
Figure 1: A lake with a warehouse on an island.
At the core of this architectural pattern is the data warehouse. To understand it, let's start from the data lake and work towards the island of information. The data lake receives raw data from external big data sources, such as clickstream, social media, and the Internet of Things (IoT), through data streams. Data scientists and business analysts (schema on read) process this raw data as needed to create various stores for analytics, machine learning, and predictive and prescriptive business applications. Timeliness and rawness of the data are crucial for illustrative computing, where delays or summarization can diminish the analytic value. While full cleansing and reconciliation may not always be feasible, providing sufficient metadata or context-setting information is essential to make the data meaningful and maintainable.
The original concept of a data lake solely focused on its role as an informational environment for analytics and data science without creating new data. However, with the rise of prescriptive analytics and machine learning, feedback loops from the data lake involving new data and models in operational systems have become necessary.
Data Warehouses and Data Lakes: A Unified Approach
Traditionally, data warehouses and data lakes have been seen as two separate and distinct technologies. Data warehouses are designed for structured data, such as customer orders and financial transactions, while data lakes are designed for unstructured data, such as social media data and sensor data.
However, in recent years, the lines between data warehouses and data lakes have begun to blur. This is due to the increasing volume and variety of data that businesses are generating, as well as the growing need for businesses to be able to analyze all of their data, regardless of its structure.
As a result, there is a growing trend toward using a unified approach to data warehousing and data lakes. This approach involves using a single platform to store all of a business's data, regardless of its structure. This allows companies to easily access and analyze all of their data, leading to better decision-making and improved business performance.
Leveraging several platforms can be useful in implementing a unified approach to data warehousing and data lakes. Some of the most popular platforms include:
- Amazon Redshift
- Google BigQuery
- Microsoft Azure Data Warehouse
- Oracle Cloud Data Warehouse
- Cloudera Data Warehouse (CDW)
These platforms offer several features that make them well-suited for a unified approach to data warehousing and data lakes, including:
- Scalability: These platforms can be scaled to handle the increasing volume and variety of data that businesses are generating.
- Performance: These platforms can provide high performance for data analytics workloads.
- Security: These platforms offer robust security features to protect sensitive data.
- Cost-effectiveness: These platforms are typically cost-effective for businesses of all sizes.
If you want to improve your business's data analytics capabilities, consider using a unified approach to data warehousing and data lakes. This approach can help you to get the most out of your data and make better decisions that will help you to improve your business performance.
Popular Alternatives for Data Management: Data Fabric, Data Lakehouse, and Data Mesh
There has been a growing trend toward using data warehouses and data lakes to store and analyze data in recent years. However, these two technologies have different strengths and weaknesses. Data warehouses are designed for structured data, while data lakes are designed for unstructured data. This can make it difficult to use both technologies together.
To address this challenge, several vendors have developed new architectural patterns that combine the strengths of data warehouses and data lakes. These new patterns include:
- Data fabric is a unified layer that sits on top of data warehouses and data lakes. It provides a single view of all of the data, regardless of its format. This makes it easier for users to access and analyze data.
- Data Lakehouse is a hybrid architecture that combines the features of a data warehouse and a data lake. It provides a data warehouse's performance and scalability, along with a data lake's flexibility and agility.
- Data mesh is a decentralized architecture that treats data as a product. Each data product is owned and managed by a team that is responsible for its data lifecycle. This approach can improve data quality and governance while reducing the risk of data silos.
Each of these architectural patterns has its own advantages and disadvantages. The best choice for a particular organization will depend on its specific needs.
Data Fabric
Pros
- Provides a single view of all data.
- Easier to access and analyze data.
- Supports both structured and unstructured data.
Cons
- It can be complex and expensive to implement.
- It may not be suitable for all organizations.
Data Lakehouse
Pros
- Provides the performance and scalability of a data warehouse.
- Offers the flexibility and agility of a data lake.
- Can be implemented using existing data warehouse and data lake infrastructure.
Cons
- It may not be suitable for all workloads.
- It may require a significant investment in new hardware and software.
Data Mesh
Pros
- Improves data quality and governance.
- Reduces the risk of data silos.
- Can be implemented using existing data warehouse and data lake infrastructure.
Cons
- It can be complex to implement and manage.
- It may not be suitable for all organizations.
Ultimately, the best way to choose between these architectural patterns is to consult with a data expert. They can help you assess your specific needs and recommend the best solution for your organization.
Conclusion
The data warehouse has played a crucial role in decision-making support for three decades, while the data lake emerged as a complementary concept a decade ago. Although they initially seemed competitive, they have evolved into equal partners, each serving different motivations. The warehouse provides reconciled and legally founded data for running and managing the business, while the lake offers a platform for storing raw data and enabling innovative analysis in an ever-changing paradigm. Recognizing these components' distinct but complementary roles is key, regardless of the specific terminology used.
The evolution of data warehouse architecture from warehouse vs. lake to warehouse and lake is a positive development. It gives businesses more flexibility and choice in storing and analyzing their data. This can lead to better decision-making and increased innovation.
As data grows in volume and complexity, a hybrid data warehouse and data lake solution will only become more mission-critical. The solutions listed above are all well-positioned to meet this need.
The recent definitional differences and implementation challenges have led to the emergence of three new architectural patterns: data fabric, data mesh, and data lakehouse. These patterns aim to merge the roles of warehouse and lake through different organizational approaches and technologies. We gain insights into these new architectural patterns by conceptualizing a data warehouse as an island of information within a data lake and considering the positioning and movement of data and function within this collaborative environment.
Many different data warehouse and data lake solutions are available on the market. Some popular examples include:
- Cloudera Data Warehouse
- Amazon Redshift
- Microsoft Azure Data Warehouse
- Google BigQuery
- Snowflake
In response to evolving business needs, a hybrid implementation can be achieved by migrating some traditional data or data marts to the data lake ecosystem, taking advantage of its advancements in multi-function analytics. Additionally, certain functions such as data preparation and archiving can be moved out of the data warehouse, extending its lifespan and reducing operational costs. Striking the right balance between data and function enables a more efficient hybrid approach.
This architectural evolution from warehouse vs. lake to warehouse and lake promises to provide business users with the much-needed cross-environment illustrative function for exploring data creatively. It also allows the warehouse environment to focus on fulfilling functional requirements, ensuring correct and consistent data compliance with business, legal, and regulatory needs. Furthermore, the integration and connection of the data lake and warehouse create opportunities for conventional and digitally transformed businesses, unlocking the potential of more data-driven possibilities.
Here are some additional benefits of using a hybrid data warehouse and data lake solution:
- Reduced costs: By combining the two technologies, businesses can reduce the costs of storing and managing their data.
- Increased performance: A hybrid solution can provide better performance for both structured and unstructured data.
- Improved security: A hybrid solution can provide better security for both structured and unstructured data.
- Enhanced flexibility: A hybrid solution can provide more flexibility for businesses to store and analyze their data.
With the continued development and adoption of these integrated approaches, organizations can harness the power of both data warehouses and data lakes to fuel their growth and drive innovation in the ever-expanding landscape of data-driven decision-making.
We Provide consulting, implementation, and management services on DevOps, DevSecOps, DataOps, Cloud, Automated Ops, Microservices, Infrastructure, and Security
Services offered by us: https://www.zippyops.com/services
Our Products: https://www.zippyops.com/products
Our Solutions: https://www.zippyops.com/solutions
For Demo, videos check out YouTube Playlist: https://www.youtube.com/watch?v=4FYvPooN_Tg&list=PLCJ3JpanNyCfXlHahZhYgJH9-rV6ouPro
If this seems interesting, please email us at [email protected] for a call.
Relevant Blogs:
Recent Comments
No comments
Leave a Comment
We will be happy to hear what you think about this post